Paper accepted in IEEE Transactions on Robotics
26.01.2024This paper presents Uni-Fusion, a universal continuous mapping framework for surfaces, surface properties (color, infrared, etc.) and more (latent features in contrastive language-image pretraining (CLIP) embedding space, etc.). This is the first universal implicit encoding model that supports encoding of both geometry and different types of properties (RGB, infrared, features, etc.) without requiring any training.
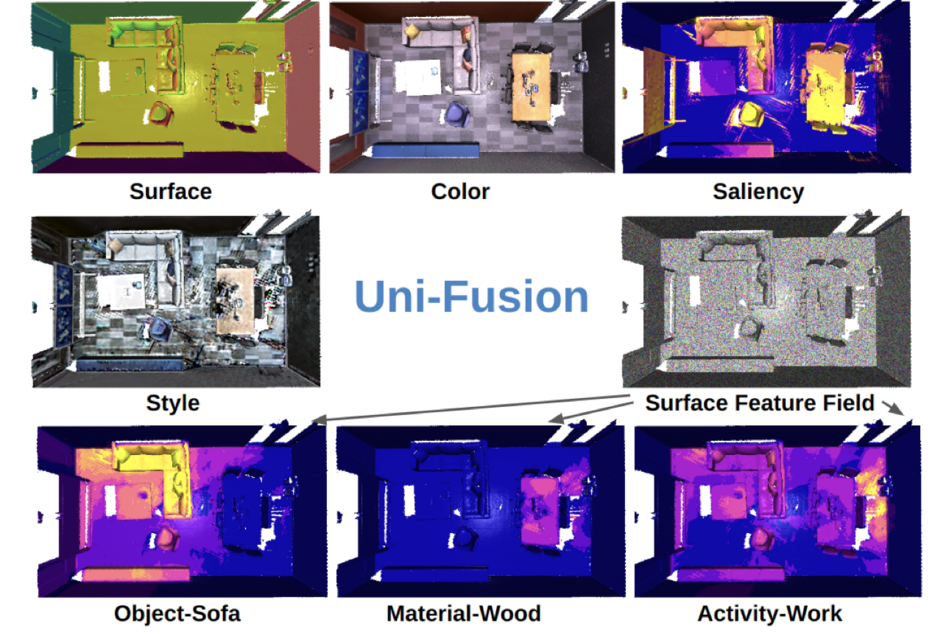
Based on this, our framework divides the point cloud into regular grid voxels and generates a latent feature in each voxel to form a latent implicit map (LIM) for geometries and arbitrary properties. Then, by fusing a local LIM frame-wisely into a global LIM, an incremental reconstruction is achieved. Encoded with corresponding types of data, our LIM is capable of generating continuous surfaces, surface property fields, surface feature fields, and all other possible options. To demonstrate the capabilities of our model, we implement three applications: incremental reconstruction for surfaces and color, 2-D-to-3-D transfer of fabricated properties, and open-vocabulary scene understanding by creating a text CLIP feature field on surfaces. We evaluate Uni-Fusion by comparing it in corresponding applications, from which Uni-Fusion shows high-flexibility in various applications while performing best or being competitive.
[Download Paper ] [Youtube]