Julian Tritscher, M.Sc.
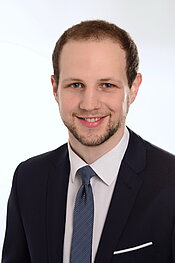
Email: tritscher[at]informatik.uni-wuerzburg.de
Projects and Research Interests
Explainable Artificial Intelligence - Anomaly Detection - Machine Learning
I worked at the Data Science Chair from 2019 to 2024. I focused on investigating the explainable detection of anomalous and fraudulent behavior in ERP (Enterprise Resource Planning) systems in the DeepScan project. Further, I worked on the AI@Knauf project, integrating machine learning approaches into industrial manufacturing processes.
The research focus of my dissertation was the domain of explainable artificial intelligence (XAI). Here I worked on providing intuitive explanations for decisions from complex anomaly detection approaches.
Teaching
Activities
- PC member for the MIDAS Workshop on MIning DAta for financial applicationS at ECML-PKDD (2023, 2024)
- PC member for the 47th German Conference on Artificial Intelligence (2024)
- PC member for the KDML Workshop on Knowledge Discovery, Data Mining and Machine Learning at LWDA (2024)
Publications
2024[ to top ]
-
“Generative Inpainting for Shapley-Value-Based Anomaly Explanation”, The World Conference on eXplainable Artificial Intelligence (xAI 2024).(2024)
-
“Data Generation for Explainable Occupational Fraud Detection”, 47th German Conference on Artificial Intelligence (KI 2024) - to appear.(2024)
-
“Benchmarking of synthetic network data: Reviewing challenges and approaches.”, Computers and Security, 145, 103993, available: http://dblp.uni-trier.de/db/journals/compsec/compsec145.html#WolfTLHS24.(2024)
2023[ to top ]
-
“Feature relevance XAI in anomaly detection: Reviewing approaches and challenges”, Frontiers in Artificial Intelligence, 6, available: https://doi.org/10.3389/frai.2023.1099521.(2023)
-
“Evaluating feature relevance XAI in network intrusion detection”, The World Conference on eXplainable Artificial Intelligence (xAI 2023).(2023)
-
“Occupational Fraud Detection through Agent-based Data Generation”, The 8th Workshop on MIning DAta for financial applicationS MIDAS 2023.(2023)
2022[ to top ]
-
“Open ERP System Data For Occupational Fraud Detection”, arxiv, available: https://arxiv.org/abs/2206.04460.(2022)
-
“Towards Explainable Occupational Fraud Detection”, Machine Learning and Principles and Practice of Knowledge Discovery in Databases. ECML PKDD 2022, Communications in Computer and Information Science(1753), 79–96, available: https://doi.org/https://doi.org/10.1007/978-3-031-23633-4_7.(2022)
2021[ to top ]
-
“A financial game with opportunities for fraud”, in 2021 IEEE Conference on Games (CoG), 1–5, available: https://doi.org/10.1109/CoG52621.2021.9619070.(2021)
2020[ to top ]
-
“Emote-Controlled: Obtaining Implicit Viewer Feedback through Emote based Sentiment Analysis on Comments of Popular Twitch.tv Channels”, ACM Transactions on Social Computing, available: https://doi.org/10.1145/3365523.(2020)
-
“Evaluation of Post-hoc XAI Approaches Through Synthetic Tabular Data”, in Helic, D., Leitner, G., Stettinger, M., Felfernig, A. and Raś, Z.W., eds., Foundations of Intelligent Systems, Cham: Springer International Publishing, 422–430.(2020)