Predictive Bridge Information Deep Learning Modell zur Anomalieerkennung
Motivation
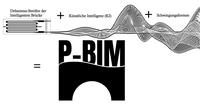
Die Problematik des Brückenmonitorings mittels sensorbasierter Überwachungsmethoden liegt historisch bedingt darin, dass zum genauen Abgleich der Messdaten mit dem Tragwerksverhalten zumeist die grundlegende Messung im Neubauzustand fehlt. Demnach können häufig nur Grundannahmen zum Normalzustand getroffen werden. Das Konzept des digitalen Testfelds Autobahn der Bundesanstalt für Straßenwesen (BASt) begegnete diesem Problem damit, dass erstmals ein neu gebautes Bauwerk im Kreuz Nürnberg mit externer Vorspannung direkt beim Neubau mit einem sensorischen Messkonzept ausgestattet wurde. Die Zielstellung des Konzeptes der intelligenten Brücke, den Status des Bauwerks zu überwachen und datenbasierte Entscheidungs-Grundlagen für ein prädiktives Lebenszyklusmangement zu schaffen wird durch das P-BIM Projekt weiter vorangetrieben.
The problem of bridge monitoring by means of sensor-based monitoring methods lies in the fact that the basic measurement during the new construction condition is usually missing. Thus, the exact comparison of the measurement data with the initial state of the structural behavior is not possible. Accordingly, often only basic assumptions about the initial state can be made. The concept of the digital highway test field of the German Federal Highway Research Institute (BASt) addressed this problem by equipping a newly built structure at the Nuremberg intersection with external prestressing with a sensory measurement concept directly during new construction for the first time. The objective of the intelligent bridge concept, to monitor the status of the structure and to create a data-based decision-making basis for predictive lifecycle management, is being further advanced by the P-BIM project.
Projektziel / Objectives
Ziel von P-BIM ist es, durch ein Deep Neural Network lokale, schadensbedingte Änderungen bzw. Anomalien am Schwingungsverhalten zu erkennen und dadurch zur Weiterentwicklung des BIM-Ansatzes beizutragen. Anvisiert wird ein datenbasiertes Entscheidungssystem zur Beurteilung von lokalen Anomalien und Schadensklassen, das zur Steigerung der Investitionseffizienz die Weiterverwertung bestehender Daten des mFunds forciert. Bei erfolgreichem Proof of Concept (POC) könnten die normative Zustandsbeurteilung durch Brückenprüfungen nach DIN 1076 um Kennwerte des Tragsystemzustandes ergänzt und ggf. der Traglastindex überarbeitet werden sowie wirtschaftliche, datenbasierte Erhaltungsstrategien und Restnutzungsdauern ermittelt werden.
The ultimate goal of P-BIM is to develop a Deep Neural Network to detect local damage-related changes or anomalies in vibration behavior and thereby contribute to the further development of the BIM approach. A data-based decision system for the assessment of local anomalies and damages is targeted, which will force the further utilization of existing mFund data to increase investment efficiency. If the proof of concept (POC) is successful, the normative condition assessment by bridge inspections according to DIN 1076 could be supplemented by characteristic values of the load-bearing system condition and, if necessary, the load-bearing index could be revised, and economic, data-based maintenance strategies and remaining useful lives could be determined.
Durchführung / Project
Zur Zielerreichung wird ein Deep Neural Network entwickelt, das die Daten der Verformung der Dehnmessstreifen (DMS) der intelligenten Brücke in den räumlichen und zeitlichen Dimensionen des Sensorgrids als Schwingungsverlauf auflöst. Die Netzwerkarchitektur wird so optimiert, dass geeignete Repräsentationen für Normalverhalten und Anomalien gelernt werden können. Überdies wird Vorwissen zu physikalischen Grundlagen der intelligenten Brücke als Information in das Netz integriert. Um die Effekte dieser Maßnahmen sichtbar zu machen wird zuletzt ein XAI (explainable artificial intelligence) Modul entwickelt, das die Ergebnisse des Netzes erklärbar und bewertbar macht.
To achieve the goal, a deep neural network is developed that resolves the strain gauge (SG) deformation data of the smart bridge in the spatial and temporal dimensions of the sensor grid as an oscillatory response. The network architecture will be optimized to learn appropriate representations for normal behavior and anomalies. Moreover, prior knowledge on physical principles of the smart bridge is integrated into the network as information. In order to make the effects of these measures visible. Moreover, an XAI (explainable artificial intelligence) module is developed, which makes the results of the network explainable and evaluable.
Projektpartner / Network partners
Veröffentlichung: BMDV - Predictive Bridge Information Deep Learning Modell zur Anomalieerkennung - P-BIM (bund.de)
Gefördert von
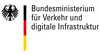
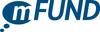